Introduction
AI presents a significant opportunity for GTM teams to achieve more and better with less, especially in a world where everything is changing fast: new technologies, shifting distribution channels, rising competition, and evolving buyer behaviors. Between Google and Microsoft cracking down on cold emails, ChatGPT reshaping how people search, social selling taking over in some industries, and rising customer acquisition costs… Traditional GTM playbooks no longer follow the same rules.
Some AI tools already reduce the gap from idea to execution - whether it’s building workflows in n8n, Clay or shipping products with Lovable. But to really stay ahead, GTM teams often need more than scattered tools. They need the right infrastructure -built around their stage, resources, and goals - and flexible enough to evolve over time
AI is already present in most organizations - sales reps and marketers use tools like ChatGPT, Claude, or Gemini for everyday tasks like writing emails, doing account research, or summarizing notes and documents. But that’s just scratching the surface. What’s still missing in many teams is a cohesive AI strategy - one that spans functions and thinks bigger than “Hey AI, write me some copy.”
There’s also growing tension between full automation and human-in-the-loop systems. While AI excels at research, enrichment, and first drafts, the best results often emerge when experts guide the process at both ends: shaping the direction and refining the output. That’s where compounding value is found: not in replacing workflows, but in reengineering them for better outcomes.
Behind all of that, data plays a central role - especially your internal data: positioning, tone of voice, customer insights, and strategic priorities. This is what takes AI output from ok-ish to great. Without that unique context, outputs risk being generic or off-mark. The more your systems are grounded in your data, the more relevant, differentiated, and unreplicable your GTM efforts become.
Of course, your approach will evolve with your stage and internal resources. When you're early and don’t have dedicated operators, it often makes sense to start with ready-to-use tools. But as your team matures and you bring on technical talent -RevOps, GTM engineers, growth-minded builders - it opens the door to a more composable stack - modular tools, custom workflows, automations, and AI agents tailored to how your team actually works - by enabling the ingestion and transformation of the right input data to maximize context.
In this article, we’ll share some practical use cases and examples that you could implement to support your GTM - from data cleaning and categorization to email personalization, account research, sales call analysis, and more.
1/ Data Cleaning
Still, a lot of basic account and contact data aren’t clean in CRMs, so a lot of people still either hesitate to use variables like {firstName} or {companyName} in their outreach because they aren’t confident about the data or don’t really care and happen to be sending some “Hello Company Inc.”, and for the most rigorous spent hours cleaning lists in Gsheets. Using GenAI to clean at scale Contact data (firstName, lastName, title) and Account data (companyName) is a huge time saver for people who need to verify this data before actioning it (in email variables, for example) and preserve prospect experience. One way of doing that is to run a simple prompt, upstream or on top of your CRM (Clay or n8n can be used), to clean all the records and safely turn them into variables you can trust in your outreach.
Here is a prompt example that transforms a company name to its commonly used version, such as from ‘National Basketball Association’ to ‘NBA’, and from ‘Tableau, A Salesforce Company’ to ‘Tableau’, etc.
2/ Data Categorization
There are tons of applications to categorize data, but here are some classic use cases that many B2B SaaS companies could use:
- Persona & Seniority: For some roles, the variety of job titles - and how creative people get on LinkedIn (cc: “Growth”, “GTM Engineer” 🙃) - makes it hard to categorize them into clear functions, teams that actually make sense for your business. That’s why training AI to recognize the relevant keywords in job titles and map them to the right persona can be super valuable. It helps you be more exhaustive and granular when building persona lists, which is key for better segmentation, personalization at scale, or data analysis.
- People nationality/language: As a French company selling abroad, a classic play is to target French people based in your target countries. The common and easiest way to do that is by checking the LinkedIn profile language - but that’s limited since many French people list ‘English’ as their profile language. That’s why looking at additional attributes like schools, previous job locations… can help generate a broader audience of people who might be French and then pass their full name to the AI to tell you if it sounds French or not. You’d be surprised how a simple “Bonjour…” at the beginning of an email (and in the preview text) increases open and reply rates!
- Activity sentiments: Your Sales & Marketing teams likely generate a lot of engagements/replies, and it’s often a challenge to score or categorize them into Negative/Neutral/Positive sentiments - something that can be super useful to drive actions from Reps, avoid potential deals slipping through the cracks, or run data analysis. Pushing the last reply sentiment at the Contact level in your CRM can be a way to do so.
- Prospects LinkedIn missions: Going beyond job titles to segment and prioritize high-fit prospects is pretty advanced to do at scale (e.g., a Marketing Manager that specifically does ABM for example) - unless you manually review each LinkedIn profile and the missions they mention in their current and past roles. A good place to start is by playing around with the keyword search in LinkedIn Sales Navigator to generate a first list of contacts mentioning relevant terms. From there, you can train AI to identify the types of missions that match your criteria.
Example: let’s say you’re selling a CRM and want to identify people who mention having implemented HubSpot in a previous role. There are so many ways to say "implemented"- you might see “set up,” “selected,” “led the implementation,” etc., which makes it a great use case for AI. However, it’s crucial to give clear guidelines on the expected output and iterate well on the prompt to avoid hallucinations. This high-quality audience will give you (i) confirmation they’re familiar with your space and one of your competitors, (ii) a signal they had enough seniority or influence to lead such a project, (iii) a scalable way to personalize with an icebreaker like: “Saw you implemented {vendorName} at {previousCompany} - curious how that experience was”.
Example of a People LinkedIn mission that could be extracted using AI to identify prospects who implemented SFDC in a previous experience.

3/ Account & Prospect Research
Some tools already automate account and prospect research, but most rely mainly on third-party data or public info such as recent news, LinkedIn profiles, etc. The problem? They lack context on your product, your positioning, and what truly matters for your prospects, as they don’t really leverage internal, first-party data - your real gold mine. What’s missing is the ability to feed AI with what makes your GTM strategy unique: relevant external signals, past Sales conversations, Marketing engagements, and internal knowledge (battle cards, enablement decks…). That’s how you bring relevance, specificity, and real differentiation - so GTM teams can craft a strong POV and stand out.
Here is an example of ‘AI Account Planner’ that centralizes data in one place, as it is often spread across multiple sources, and provides Reps with a clear and structured view to digest the information - rather than navigating through different tools and having to click through various CRM records, sections, websites, etc. The different sections are:
- Overview: firmographic data (from internal CRM)
- Documents & Summaries: 10-K or 10-Q reports available + the ability to input other documents with AI extracting/labelling the relevant information only
- Business Units: identify relevant subsidiaries (Business Units, Geographical Split…) - useful to spot gaps & ensure completeness of territories
- Contact Recommendations: highlight top prospects to quickly spot whitespace & drive action on account learning
- Recent News: relevant to the business and value proposition
- Gong Calls: previous call summaries and highlights

Account Plan highlighting a point of view based on news, internal calls, 10-K reports, and recommended contacts for each use case.

Tip: To save credits (and money), don’t generate an Account Plan for every account in your CRM. Instead, consider adding a trigger in Salesforce - like a button to generate it on demand, or automating the workflow so it only runs after a discovery call is booked, for example. The account research features of Tamtam.ai or Pocus can serve as plug-and-play alternatives.
4/ Email Generation
Data + AI + human brain for hyper-targeted outreach
With AI and the latest tools on the market, capturing valuable data is easier than ever. However, two key challenges remain:
- providing the Sales team with the right level of information in the right system
- making it easy to take action (removing friction between “I want to reach out” and actually hitting send)
It’s all about striking the right balance between data and human intelligence - to connect the dots, verify insights, and adapt the messaging for the most effective outreach.
Here is an example of internal tool to:
1️⃣ Let the Sales team surface key contacts in their territory with:
- Smart views (aka Sales plays) such as ‘reconnect with lost opportunities’, ‘my contacts with marketing engagements… to adopt the best practices of top-performing Reps
- Tailored contact level ‘Reasons to Engage’ based on Insights (such as new position, previous user…), Marketing engagements (attended an event, downloaded an eBook…), previous sales conversation (cold call from a past Rep, prospect included in a past demo), etc.
2️⃣ Support them by crafting a personalized email based on the above ‘Reasons to Engage’ and let them provide more context in the prompt so they can add metadata that wasn’t captured in the current engine or guide the prompt toward the right approach, tone of voice, etc.
Rather than a fully automated model, here we focus on a hybrid approach where the Reps can (i) guide the prompt based on recommended reasons to engage as we still believe the human brain is best to pick the most relevant information and (ii) verify and modify the content before pushing it to the Sequence, giving Sales Reps full control.
The data has been anonymized and modified to protect the confidentiality of the prospects
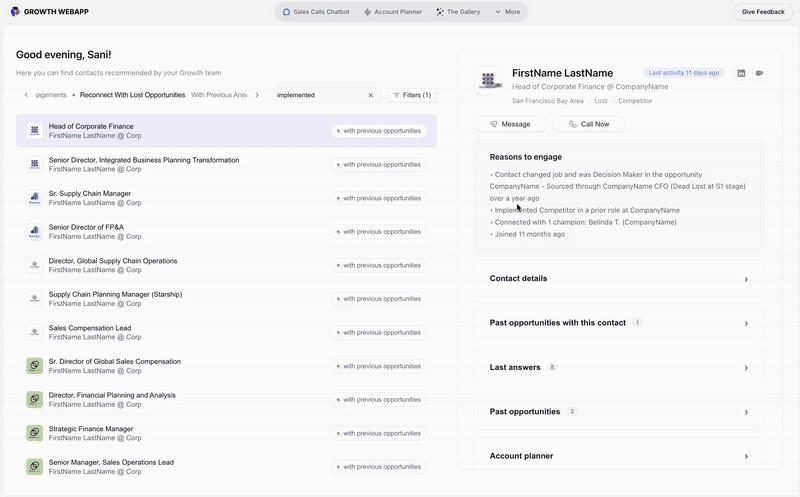
AI-generated replies for pre-event acquisition
Using outbound to drive registrations for events (webinars, product tours, in-person sessions…) is a repeatable way to engage prospects through a soft, low-pressure approach - especially those who aren’t ready to speak with Sales yet.
From our tests, we’ve seen better results when we don’t include the registration link in the first message. Instead, we ask if the event could be relevant for them or if they’d like to receive more details. It sounds more natural, improves deliverability, and tends to generate more replies.
The downside: the registration is not self-serve, which means we have to manually handle hundreds of replies for each campaign - and that becomes a real operational challenge as we scale.
To reduce the workload on people managing those responses, you can set up an AI-powered workflow that suggests a reply template based on the prospect’s message - whether it’s a no, a request for more info, or someone asking for the recording. The idea is to make it easy to modify and push a relevant answer into your Engagement tool, with light manual editing or personalization when needed.
Example of an AI-generated response to a prospect’s reply about an event invitation

5/ Conversational Agents
Most companies already use a conversation intelligence tool like Gong, Modjo, or Attention to record and analyze sales calls. Building a conversation agent on top of these call transcripts opens up a new layer of insight, enabling you to search and query across all calls to identify broader patterns. The goal isn’t just to focus on individual deals, but to aggregate conversations at scale and unlock strategic learnings.
For example, you can:
- Spot recurring pain points or patterns based on attributes like industry, company size, competitors used, or persona
- Gather competitive intel to understand why you win or lose against certain players - especially useful for Product Marketing
- Bridge the gap between your marketing messaging and how prospects actually talk - surfacing killer quotes you can reuse in cold emails or sales enablement. This is especially helpful when you're not the persona you're selling to and need to nail the right tone, wording, and verbatim.
Example of a conversation to learn more about specific pain points related to a use case and find patterns and prospects’ quotes.

Conclusion
GTM strategies are evolving fast, and adopting AI isn’t a nice-to-have anymore - it’s the baseline. While many teams are still discussing roadmaps or searching for the perfect tool to buy, some of the most impactful solutions are already being built in-house by those closest to the problems. Whether it’s a quick automation, a scrappy workflow, or a GPT-powered agent, internal builds can sometimes move faster than waiting on the ‘perfect’ vendor (who might not even exist yet or is in stealth mode as we speak!) selection or release.
Again, the solution depends on the current state of your GTM, the biggest pain, the stage of the company and its resources. It doesn't have to be over-engineered. Instead, when internal resources are lean and you don't have the human resources and bandwidth, it's easier to move with plug-and-play tools. These will help you go at the expected speed without complex and custom workflows. But as you grow, your strategy can (and probably should) evolve. Your organization isn't the same, and your processes are... yours. This means it becomes harder for (rigid) tools to tick all your boxes. With this in mind and more resources, it makes more sense to choose a compostable stack built around internal context, modular tools, and flexible workflows. This becomes a huge enabler for your GTM team.
At the same time, many Sales Reps & Marketers are already experimenting on their own, like building personal GPTs. As a RevOps or Growth team, one of the highest-leverage things you can do is to spot and centralize those innovations - turn them from shadow tools into shared capabilities, and help others benefit from what’s already working.
That said, it’s easy to get attached. Just because something was built internally doesn’t mean it has to stick around forever. If a better external solution comes along - one that scales better, requires less maintenance, or improves usability - that’s a good reason to evolve. It’s not a binary choice between building or buying. It’s a mindset shift: prototype quickly, remove friction, and stay agile. When the pain is high and the external options are clunky, build.
Because at the end of the day, the goal isn’t to build tools - it’s to generate pipeline and revenue, speed up decisions, and help GTM teams focus on what they do best: engaging the right people, at the right time, with the right message. It’s less about making the perfect call and more about keeping momentum.
Chances are, you’re already building. The question is whether those efforts are scalable and supported - or quietly buried in someone’s personal folder. With the AI revolution just beginning, staying curious, adaptable, and collaborative might be the real GTM advantage.
🧰 There are plenty of tools emerging in the AI & GTM tech space. Here are a few we recommend taking a look at:
- Cargo → AI workflows
- n8n → AI agent feature for workflows
- Actively → Account planning and AI-powered outreach
- Copy.ai → AI workflows
- Unify → capture signals, build plays and engage
- Attention → AI agents for Sales calls
- dust → build AI agents on top of your internal tools
- Pocus → AI agents that monitor accounts
- Tamtam.ai → AI agents for Meeting Prep, Account Mapping, Org Charts…
- folk → AI-driven CRM
- Topo → AI-SDR with humans driving the strategy
- Userled → AI-personalized marketing experiences
👋 Thanks for reading! Subscribe for free to receive new posts.